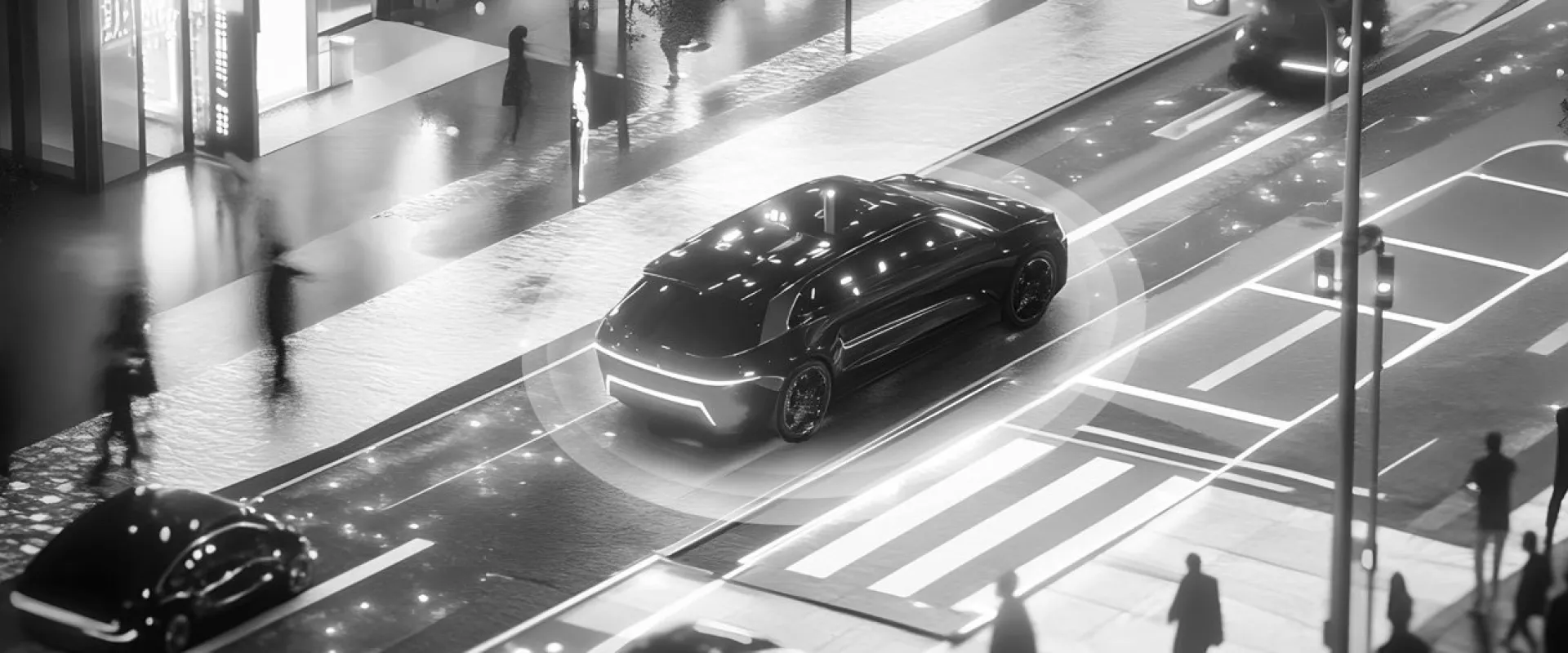
Smart cars, safe roads: how semiconductor technology makes traffic safer
The automotive industry is rapidly evolving, with safety and sustainability gaining increasing priority.
A wide range of semiconductor innovations can help us meet the ambitious goal of zero traffic fatalities. Sensors, radar systems and AI processors allow cars to process vast amounts of data from their surroundings and make real-time decisions that can avoid accidents.
One of the most promising advancements in making roads safer is the integration of advanced driver assistance systems or ADAS, which rely on an array of semiconductor technologies to help vehicles detect hazards, prevent accidents, and minimize human error.
Data-driven decisions with ADAS
Over 90% of crashes are caused by human error. A staggering statistic. Drivers may misjudge oncoming traffic, are distracted, or simply too tired to respond immediately to an unexpected situation. They may also take the wrong decision misled by poor road design and dangerously high speed limits.
Advanced driver assistance systems or ADAS tackle this road safety issue by making data-driven decisions that are faster and more precise than a human could manage in real time. ADAS act as the car’s ‘eyes and ears’, relying on a combination of sensors, cameras, radar, and AI-driven processors to monitor the vehicle’s surroundings and assist drivers in avoiding potential hazards. ADAS technologies can help prevent collisions by issuing warnings, applying emergency brakes, or even taking over certain driving tasks when necessary.
These systems can detect vehicles in blind spots, assist with lane-keeping, and even offer automated parking solutions, all powered by semiconductor innovations.
Sensor fusion: capturing more information
No single sensor can provide a complete picture of a vehicle's surroundings.
- Cameras, for example, excel at capturing detailed images but can struggle in low light or harsh weather conditions.
- Radar, on the other hand, can detect objects through fog or rain but lacks the resolution to identify whether an obstacle is a person, a vehicle, or something else.
- Lidar, which uses laser pulses to measure distances, offers high-resolution 3D mapping of the vehicle’s surroundings, but is more complex, cumbersome, and expensive.
This is where sensor fusion—the process of combining data from multiple sensors—plays a vital role in ADAS.
Sensor fusion allows a vehicle to create a comprehensive, 3D view of its environment by merging inputs from radars, cameras, and other sensing technologies. By leveraging data from these different sources, the system can build a clearer and more accurate representation of the road ahead, minimizing blind spots and improving real-time decision-making.
One innovative approach is cooperative radar-video sensor fusion, where radar and cameras work together to enhance object detection. For instance, if the radar detects a reflective metal object, the camera system can adjust its settings to better identify what the object is.
This fusion of low-level data between sensors leads to greater detection accuracy, particularly in challenging conditions, such as fog, rain, or crowded urban environments.
Researchers at imec and UGhent have shown that especially in difficult circumstances (poor visibility, crowded areas, objects appearing from occluded areas) cooperative fusion of inputs can improve pedestrian detection by as much as 15% compared to traditional detection methods.
AI and machine learning: making split-second decisions
To process and act on the vast amounts of data collected by sensors, ADAS applies AI and machine learning. These technologies analyze data from cameras, radar, and lidar, allowing vehicles to not just observe, but also predict and react to potential dangers in real time.
AI algorithms can recognize patterns, like a pedestrian stepping into the street or a cyclist changing lanes. By using deep learning models, ADAS systems can classify objects (cars, pedestrians, cyclists) and anticipate their movements, reducing the likelihood of accidents.
Much like human drivers who rely on experience, AI continuously learns from the data it gathers. This enables ADAS to adapt to new situations, improving its performance as it encounters a greater diversity of road conditions and driving environments.
The AI models and algorithms can also be further improved and refined. Today’s AI engines are often trained to detect as many vulnerable road users as possible. But is it always necessary to detect a pedestrian who has already crossed the road fifty meters ahead? Computational resources should perhaps focus on imminent threats, like pedestrians or vehicles that are within the car’s projected path. The system could learn to prioritize critical tasks, such as identifying a pedestrian in a car’s path, over less immediate information.
Overcoming performance and energy challenges
Achieving seamless, real-time performance that can deliver on the promise of fully autonomous driving technologies is still a challenge today. One of the most pressing issues is the need for high bandwidth and processing power to handle the massive amounts of data generated by different sensors. These systems must process all data quickly to make split-second decisions—without draining a vehicle’s power supply, especially in electric vehicles.
For ADAS to operate effectively, semiconductors must balance high performance with energy efficiency. Advanced AI algorithms that power ADAS require heavy data processing, and monolithic chips are not optimized to handle such workloads efficiently. This is where innovations like AI accelerators and specialized automotive chips come into play. These chips are designed to process sensor data in real time while consuming far less energy, making them ideal for the high-performance, low-power demands of modern vehicles.
One promising approach to overcoming these challenges is to use chiplet-based architectures. Chiplets allow for modular and scalable chip designs, offering a flexible solution to balance high performance with energy efficiency in a way that monolithic chips struggle to achieve. By splitting complex processing tasks across smaller, specialized chiplets, vehicles can process data more efficiently and reduce power consumption.
Another challenge is latency, or the delay between data collection and decision-making. In complex traffic situations, even a split-second delay can be critical. By reducing this lag, vehicles can react more quickly to unexpected obstacles, improving overall safety. The solution lies in developing low-latency, high-bandwidth integrated circuits that can transfer large data streams from sensors to the vehicle’s central processing unit almost instantly.
Semiconductor researchers are working to create next-generation chips that combine power efficiency with the processing speeds needed for advanced AI systems, ensuring that vehicles can handle complex environments while maintaining long battery life.
Semiconductor innovations for safer roads
As semiconductor technology continues to evolve, the future of ADAS and road safety looks increasingly promising. The next phase of automotive safety will focus on further refining sensor technologies—making them smaller, more cost-effective, and more accurate. At the same time, AI algorithms will continue to improve, allowing vehicles to not only react to immediate dangers but also anticipate potential hazards by predicting the behavior of pedestrians, cyclists, and other drivers.
Achieving these ambitious goals will require continuous collaboration between the automotive and semiconductor industries. That is why initiatives like star bring car manufacturers and technology leaders together to ensure that semiconductor advancements not only meet the safety and performance demands of the automotive sector but also support the industry’s broader sustainability goals. These efforts show we can create safer roads and cleaner cars, improving mobility without compromising the health of people or the planet.